Data Science Aids Lead-Service-Line Inventory And Replacement Programs

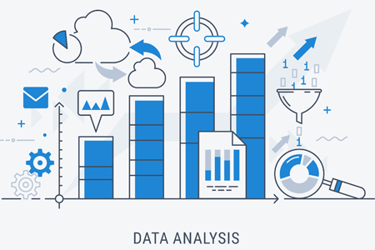
A white paper recently released by the Association of State Drinking Water Administrators (ASDWA) provides insights on how water utilities can better use data to manage uncertainty around remaining lead-service-line (LSL) customer connections. The document, Principles of Data Science for Lead Service Line Inventories and Replacement Programs, represents the organization’s commitment to making information accessible to assist state program administrators in protecting public health. It was developed for ASDWA by BlueConduit, a water-infrastructure analytics consulting company.
The Challenges Of LSLs
Concern around the U.S. EPA’s Lead and Copper Rule (LCR) is particularly timely because the long-term LCR revisions posted on November 13, 2019 are currently under review for anticipated release in late 2020. The proposed revisions include a series of significant monitoring, treatment, and remediation challenges, which generated a wide range of concerned responses before the public comment period closed on February 12, 2020.
One of the more challenging concerns is in generating accurate inventories of LSLs for regulatory reporting and remediation efforts. Doing so can be hard to achieve because of inadequate recordkeeping — especially with older properties. An added reason for the importance of identifying trouble spots is that it can also affect access to potential funding for help with LSL replacement.
The Value In Good Data Practices
The ASDWA white paper underscores how each of a state’s water utilities can take advantage of good data science to help them implement the finalized rules when they are ultimately released and to improve their ability to forecast properties likely in need of LSL replacement.
The document reflects experience with methodologies used to support LSL replacement efforts in Flint, MI. It focuses attention on five key principles, each of which is accompanied by notes on topics specific to water utilities and their administrators and on lessons relevant to that principle learned through the City of Flint’s experience. Charts and graphics are also included to illustrate those relevant principles, which include:
- Clean Data Management And Organization. Regardless of the quality of historical records or other sources of information, it is important to label data in the new database or spreadsheet accurately and consistently, in order to maintain its value for reliable predictive modeling. If data exists from older manually kept notes, it must be digitized in order to be helpful as part of a digital analysis.
- Not Accepting All Historical Records As Truth. Most utilities know from experience that the ‘as-built’ materials and locations of water-distribution infrastructure are not always the same as the ‘as-documented’ details. A confusion matrix from the City of Flint’s experiences, as displayed in the report, shows how much that reality can differ from the historical record.
- Conducting A Representative Randomized Sample Of Service Lines. This principle underscores the importance of using representative, random sampling to be indicative of the size and location of potential LSL problems across the entire utility. Proper data-science practices will enable a utility to project a statistically valid representative map of LSL locations despite only having to verify a fraction of ‘unknown-material’ service-line locations.
- Transparency In Public Outreach And Reproducibility. Because the topic of lead in drinking water is such a hot-button issue, explaining how predictive modeling works can ease resident concerns about properties classified as having ‘unknown’ service-line materials. It can also bolster public support for LSL replacement programs. The document also identifies resources for helping utilities communicate such issues with the public.
- Accuracy On Held-Out Samples. Finally, the document cites the importance of using a hold-out sample set to substantiate the validity of model performance. A common approach could be using 75 to 80 percent of the data to build models and using the remaining 20 to 25 percent of held-out data to validate the performance of those models after they have been refined. In Flint, taking such an approach yielded an average 70-percent hit rate — where 8,833 targeted service lines yielded accurate predictions of lead and galvanized materials at 6,228 of those locations over a two-year period of 2016 and 2017. In some months, that hit rate rose as high as 90 percent.
Where Do We Go From Here?
While having accurate forecasting of problematic service-line materials based on data analysis by age of building, geography, historic notes, etc. is just one factor in choosing the most likely locations to excavate, it can help utilities make more informed decisions when compared against other factors.
To help drinking-water administrators get more familiar with the opportunities for better LSL decision-making through data science, the ASDWA white paper also includes multiple links for support resources and for more in-depth write-ups of data-science approaches applied in Flint.