The Role Of AI In Reducing Water Contamination Risks
By Ainsley Lawrence
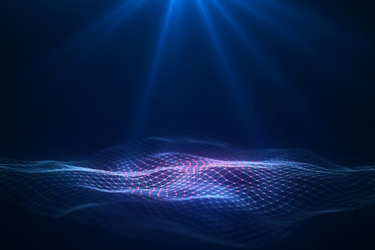
Insights gained from artificial intelligence (AI) herald a high-water mark for public protection.
Water isn’t optional. It’s the foundation of life, industry, and civilization itself. But keeping it clean? That’s a constant fight. Contaminants don’t announce themselves. They slip in through failing infrastructure, industrial runoff, and aging water-treatment systems, often going undetected until the damage is done.
That’s where AI is rewriting the rules. Predictive analytics is flipping the entire system from reactive to proactive. Instead of waiting for contamination to be discovered, utilities and industries can predict where, when, and how it’s likely to happen.
This is more than an upgrade. It’s a revolution. And in an industry where prevention is everything, predictive analytics is the tool that finally puts water managers ahead of the problem.
The Power Of AI And Data Science In Water-Quality Management
Water management has always been a battle against the unknown. Contaminants don’t follow schedules, and traditional testing methods only provide a snapshot of conditions at one moment in time. Data science and AI1 change that. They turn raw data into foresight by observing patterns, detecting problems, and more.
How AI Detects Contamination Before It Happens
AI thrives on patterns. It processes vast amounts of real-time data from sensors, historical trends, weather reports, and infrastructure conditions to detect contamination before it escalates. A shift in pH? That could mean a chemical spill. A sudden chlorine drop? A microbial breach might be forming. AI anticipates anomalies, giving water managers a critical window to take action before the contamination spreads.
And the scale is massive. AI models can monitor thousands of data points at once, spotting weaknesses in aging infrastructure, identifying pressure drops that indicate pipe damage, and even predicting seasonal contamination risks based on environmental conditions. It’s the closest thing the industry has to a crystal ball — and it’s already proving its value.
Comparing Water-Quality Risks: Well Water vs. City Water
All water isn’t equal. Well water and city water2 face entirely different challenges, but contamination is universal. AI helps both, tailoring predictive models to match their unique vulnerabilities.
City Water: A Complex Network With Hidden Risks
Public water goes through treatment, but that doesn’t guarantee safety. Infrastructure is aging, chemical byproducts form in pipes, and contamination events can start anywhere in the system. A broken main can send rust and lead into drinking water. A treatment failure can allow bacteria to slip through unnoticed.
Predictive analytics gives utilities the ability to see weak points before they turn into crises. AI models analyze distribution networks, flagging inconsistencies in pressure, chemical levels, and flow rates. A sudden shift in turbidity? A pipe might be failing. A pattern of bacterial growth near a plant’s output? The filtration process could need adjustment. AI takes what used to be guesswork and turns it into actionable intelligence.
Well Water: A System Without Oversight
For well owners, water safety is entirely self-managed. There’s no centralized testing, no automatic filtration, and no backup system when contamination happens. Agricultural runoff, heavy metals, and naturally occurring toxins like arsenic can all seep into groundwater without warning.
That’s why AI-driven monitoring is a game-changer for private wells. Predictive models can track environmental factors like rainfall, soil saturation, and nearby land use to assess contamination risks. If conditions indicate an increased likelihood of bacterial growth or chemical infiltration, AI can recommend testing before problems arise. It’s a level of protection that owners have never had access to before.
Predictive Analytics In Action: Real-World Applications
AI in water management is already being deployed to stop contamination, extend infrastructure life, and cut costs.
One major breakthrough is AI-driven pipeline maintenance. Instead of reacting to breaks, utilities are using predictive analytics to pinpoint stress points in the system before failures occur. By analyzing pressure fluctuations, material degradation, and past leak patterns, AI can forecast which pipes are most likely to fail and recommend preemptive repairs. The result? Fewer emergency shutdowns, lower maintenance costs, and significantly less water waste.
Another game-changer is real-time microbial risk assessment. AI models continuously monitor microbial activity, using environmental conditions, temperature fluctuations, and historical contamination trends to predict when bacterial outbreaks are most likely. Instead of waiting for an outbreak to be detected, treatment adjustments can be made before water quality is compromised.
These aren’t small improvements. They’re industry-shifting changes redefining how water safety is managed.
Why Analytical Thinking Still Matters in AI-Based Water-Quality Solutions
AI is an incredible tool — but it isn’t flawless. Predictive models are only as good as the data they’re trained on. A faulty sensor can send a flood of false alerts. A model built on outdated trends might miss emerging threats. That’s why human oversight is still essential.
The Role Of Human Expertise
AI can tell you something’s off, but it can’t always explain why. It might flag a sudden pH drop, but is it an actual contamination event or just a temporary fluctuation? That decision still requires experienced professionals who can interpret the data and take the right action.
That’s why the best water management strategies don’t rely on AI alone. They integrate predictive analytics with human expertise, ensuring that AI-driven insights are used effectively rather than blindly followed.
Preventing AI Misinterpretation
Even the most advanced AI can make mistakes. A malfunctioning sensor might misread chlorine levels, triggering an unnecessary alarm. A sudden water-pressure shift might be misclassified as a break when it’s actually a routine demand fluctuation. That’s why utilities need a system of checks and balances: AI-driven insights combined with expert verification.
Predictive analytics isn’t about replacing water professionals. It’s about giving them better tools, faster insights, and a level of foresight that was previously impossible.
Conclusion
AI-driven predictive analytics is a fundamental shift in waterquality management. Instead of reacting to contamination after it happens, utilities and industries can now predict and prevent it entirely.
The advantages are undeniable: faster detection, smarter intervention, and stronger infrastructure. But technology alone isn’t enough. AI provides the data, but human expertise makes sense of them. The future of clean water is about the perfect balance of innovation and experience. And with that balance, the industry is moving closer than ever to truly safe, reliable water.
References:
- https://onlinesoe.tufts.edu/blog/where-data-science-and-ai-meet-business-applications/
- https://www.culligan.com/blog/well-water-vs-city-water
About The Author
Ainsley Lawrence is a freelance writer who lives in the Northwest region of the U.S. She has a particular interest in covering topics related to tech, cybersecurity, and robotics. When not writing, her free time is spent reading and researching to learn more about her cultural and environmental surroundings. You can follow her on Twitter @AinsleyLawrenc3.