The Devil Is In The Detail: The Role Of Digital Twins In The Water Sector
By Jacob Tompkins and Julie King
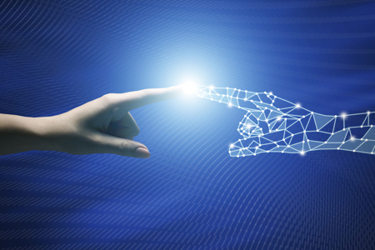
Aging infrastructure, asset maintenance, increased flooding and water scarcity, energy costs, climate change concerns, the need for flexible adaptation of assets, the need for increased resilience of new and extant assets, social issues around water costs and affordability, water quality, pollution and how to harness the benefits of the circular economy, and how to balance conflicting demands for water from different sectors — these are just some of the hot topics in water the moment. And cutting across all of these is the concept of digital water.
Digitization of water is not just a buzzword. It is already starting to have real financial and operational impacts in the water sector.
But what is digital water, and what does it practically mean for water utilities and other water stakeholders?
Digital water relates to the gathering and use of data and virtual assets.
Digital twins are part of this digital water revolution.
Digital twins are the virtual versions of real assets. Essentially, they are virtual models that mirror the behavior of the real thing.
But beyond that, there are a lot of definitions of a digital twin.
‘Data Twin’ vs. ‘Digital Twin’
In its most basic form, a digital twin is a digital schematic of a system. Imagine a big wall of data, all flashing lights and numbers and arrows, in a water company HQ, showing how much water is in each part of the system; which pumps are operating, what are the flow rates, what are the water levels and ullage of different components, and loads of other system information. This is a digital representation of the actual real system. This data is based on readings from a variety of sources ranging from user reads to real-time sensors. It could be described as a digital twin. But other than presenting system information and letting people know what’s going on, it’s not much more use than a business dashboard.
In more detailed cases, digital twins are data models that use real-time input data from sensors or SCADA to develop a range of input-output responses — e.g., if a plant has this input, it will produce this output. But these versions assume data stationarity and are constrained by the datasets they have already seen. You could argue that this is a sophisticated simulation model rather than a digital twin.
There are then digital twins that use algorithms to spot patterns in performance data and can make decisions or provide alerts based on these patterns. But in fact, these are actually the digital twins of the people who have operated the plant for 35 years and know exactly how it responds to certain circumstances, rather than a true digital twin of the system. And they still require a high degree of human interpretation and intervention.
All of these types of digital twin are good at presenting what is happening now and at ‘hind-casting’ and simulating previous scenarios, and providing optimization or predictions based on events they have already seen. But they can’t be used to model alternative or unexpected scenarios.
We would suggest that these are data twins rather than digital twins. The data twins are useful, but they can’t go beyond the datasets they are fed.
Real Digital Twins
In our view, there are two ways to build a real digital twin:
- Use historic input-response data and combine this with external datasets and advanced machine learning or AI systems, which can extrapolate from a range of existing data to model new situations and deal with new contaminants or processes. This type of digital twin will be able to offer an AI model of the real system. Or,
- Go back to first principles of chemistry, physics, biology, and hydrology and model the actual processes that occur within the system. For example, in the case of a wastewater treatment plant, this would be the physical, chemical, biological, and hydrological processes and technologies within the plant. This approach means that the digital twin will be an exact virtual model of the real physical system.
AI Augmented Data Model vs. Data Twins
The AI augmented data model or the physical facsimile model is a true digital twin as it can emulate and simulate. You can use these real digital twins to run different scenarios or to optimize multivariate optimization problems.
The augmented AI data twins and physical facsimile digital twins are also much more flexible and portable than the data twins. A good way to compare real digital twins and data twins is to think of the real digital twins as Lego models (other building block brands are available in case there are any toy lawyers reading). The data twins are detailed models of a toy house or car, which look great and can be played with. But the Lego model can be taken apart and added to and rebuilt into a castle or unicorn, because it is made of fundamental building units. Likewise, the data twins are great at representing a single water treatment plant. But you can’t add to the plant, take in new or alternative information, or apply the model to a different plant. On the other hand, the true digital twins are flexible enough to be added to or to be completely reconfigured to represent a different works (or treatment plant) because they are based on the fundamental principles of the physics, biology, and chemistry of the system.
Practical Examples
A couple of examples of AI augmented data twins or first principles-based digital twins are SCUBIC and Waterhound solutions.
SCUBIC creates real-time digital representations of water distribution networks. These digital twins use AI processes to combine real-time data from the network combined with external data such as historical water demand profiles and forecast weather data to simulate the amount of water required in each part of the network and to optimize pumping schedules to meet these demands. This leads to energy savings of around 10 percent or more. It also enables system engineers to design network extensions and to simulate future demand and to balance multivariate decisions around CAPEX, OPEX, energy use, headroom, resilience, asset life, etc. This is a true digital twin and is being used by several utilities to manage networks.
Waterhound Futures’ software creates robust, physically based digital twins for water and wastewater treatment plants. In the case of wastewater, they have built a database of modules that replicate the physical, chemical, biological, and hydrologic processes for over 800 input parameters and 300 treatment processes. These modules can be combined to build any configuration of plant and to model any range of scenarios, even in the absence of any client data. This means Waterhound’s software can also be used to simulate the performance of new plants even before they are built. Once data is present, it can be used to further optimize the performance of the digital twin, and the use of machine learning or AI can drive this even further. This means Waterhound’s software system has the flexibility of a physical-based digital twin with the constant updating of a data twin. The system has been used extensively in the food, drink, oil and gas, and manufacturing sectors with OPEX savings of 8 to 38 percent.
Advantages Of A Real Digital Twin
Digital twins represent the interface between the real and virtual data worlds. But utilities and companies must realize that the term “digital twin” is used very loosely and should look for real digital twins which can provide intelligent infrastructure modeling.
Some of the advantages of a real digital twin are:
- They behave in the same way as the real thing, so you can visualize and optimize the energy demands of a water distribution network before building the real asset.
- You can play games with your digital twin. ln the same way you can drive a Mario Kart like a madman, you can overload your digital sewage treatment plant with rainwater and loads of different contaminants and see how it behaves, which you could never do in real life.
- You can quickly run multiple scenarios with your digital twins. This enables the optimization of multivariate problems, which would be almost impossible with a real asset.
Real digital twins enable companies and utilities to monitor asset health, manage incidents, simulate and predict behavior, analyze previous performance, design new infrastructure, assess asset and network resilience to different scenarios, verify regulatory compliance, and monitor whole assets in real time. It enables companies and utilities to test scenarios in the virtual world before committing funds or building real-world assets. It enables the impact of changes in legislation (for instance discharge consent limits) to be modeled, and it means utilities and companies can play games with virtual assets to see the impact of different operation regimes on CAPEX, OPEX, energy use, compliance, headroom, or asset life. Real digital twins give companies and utilities control over asset management.
Jacob Tompkins, OBE, is cofounder and CTO of The Water Retail Company, London. Jacob is a qualified civil engineer with a degree from University College London and a MSc in hydrogeology from Imperial College London. Jacob was an environmental adviser to the Nation Farmers’ Union and a water resources and water efficiency specialist at Water UK prior to founding Waterwise in 2005, which remains the only not-for-profit organization for water efficiency in the UK. Jacob has been actively involved on EU Commission water sector committees and developed the UK’s first formal Ofqual-approved water efficiency qualification. Jacob is a Board Advisor to SCUBIC and became a Board Advisor to Waterhound Futures after seeing a presentation of Waterhound’s software.
Julie King, Managing Director, Waterhound Futures Limited, has 20-years of experience working for family offices in vetting, acquiring, developing, and commercializing small companies and start-up with digital water and clean energy technologies. She also worked for 7 years as a consult to both corporations and international conservation organizations in impact investing and in the nexus between businesses understanding the need to integrate more stringent environmental standards in operations and not-for-profits moving towards a more market-driven approach in finding innovative solutions to solve global environmental challenges. Ms. King has a law degree from Queen Mary, University of London.