SWAN Corner: Distributed Monitoring Unlocks The Value Of Data, Delivers Quality And Accuracy
By S.R. Shelley1, P. Dickenson1, L. Keogh1, S. Tait2, K. Horoshenkov3
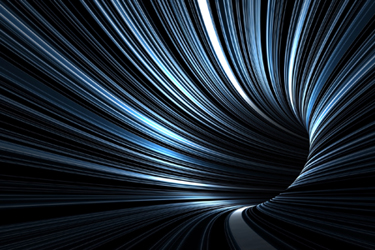
Raw sewage enters our rivers, lakes, and oceans at an alarming volume and frequency. This is a problem faced around the world. In some locations, great strides are being made to tackle this through infrastructure investment, often as a result of public and regulatory pressure, and because we all know that we need to do better.
To really make an impact and improve wastewater collection network performance, we need to understand what is happening within those networks in real time. This is important not just at pumping locations or typically every 100km [~62 miles] or so where we have a discrete monitor. Only if we know what is happening in real time can we learn to predict and optimise in real time.
Creating a digital twin of our existing wastewater infrastructure allows us to do this.
Digital twins enable network operators to monitor what is currently occurring and predict what will happen in the system. Having both these capabilities allows for the smart allocation of resources and minimises the risk of undesirable events. To create a digital twin, ‘dense contiguous data’ about the system is required. By this we mean data of a high enough resolution such that the relevant spatial and temporal length scales are well represented. For wastewater systems this means spatial resolution to a pipe length or better and timeseries data every minute. Such data is not currently available to sewer network operators but can unlock substantial savings and performance improvements.
To deliver such a digital twin we need a new state of the art infrastructure which can intelligently monitor and predict wastewater patterns. This will also allow a shift towards a proactive approach with automated operations. To achieve this new state of the art infrastructure we have several options.
Option 1: We build a lot of new networks — not really an option for obvious cost and carbon implications.
Option 2: We increase the density of our traditional spot monitors. Spot monitors are discrete sensors that provide data at a single spatial location, usually just flow rate or depth. Each one requires its own power supply and communication link, limiting scalability and making such systems vulnerable to latency and failure. To get the desired resolution we would need many sensors, spaced at relatively short intervals, perhaps even one in every chamber. Hence this option tends to be expensive and cumbersome. Even with thousands of spot monitors, most of the wastewater network will be invisible meaning the behaviour of many areas can only be determined through inference, reducing the overall accuracy and effectiveness. Machine learning and artificial intelligence can enhance this inference but are fundamentally limited by the quality and density of the input data. For example, at the flow velocity in a sewer, it can take many hours for information, such as the presence of a blockage, to propagate to the nearest spot monitor.
Option 3: We use a continuous measurement system. This provides full coverage of the wastewater network and minimises the need for any inference. Using fibre optic technology, we can also measure a wide variety of parameters with a single deployment including flow rate, depth, inflow/infiltration and detect blockages. Distributed fibre optic sensors operate by sending laser pulses down the length of a conventional optical fibre configured to act as a sensor. These pulses interact with natural imperfections in the fibre to produce backscatter whose properties such as amplitude, frequency, and phase are affected by the temperature and strain experienced by the fibre. By measuring the properties of the backscatter, the conditions around the fibre can be measured and, by time of flight, the location of each measurement point can be found. This provides a map of the flow conditions experienced within the entire collection network.
Using robots such continuous measurement systems can be permanently installed in either new builds or retrofit into existing systems and then can generate data every few metres over many kilometres of network. It is thus highly scalable. A continuous monitoring system only requires a single power source and communications point located outside the wastewater network.
Sometimes called a sewer nervous system, this can be used to produce the required high-quality, dense data that a smart city needs. As the wastewater network is not an isolated system, other data sources, such as rainfall, can be integrated into the algorithms to produce accurate predictions of the behaviour and needs of the wastewater network. By using historic data, the predictions of the system can be validated to ensure their accuracy. Equally, underlying trends of the system can be identified and accounted for. Machine learning and AI are used to interpret the large data volumes such a system produces.
The result is delivery of widespread actionable insights, allowing a shift from reactive to proactive operations. Such a shift has been shown to substantially reduce operational costs in other utilities such as power distribution and telecommunications. It also drives higher environmental performance through enabling of active management to reduce CSO spills and basement floodings. Through such next-generation smart networks, operators and municipalities can achieve the standards set by regulation and enforcement action at lower cost than simply building additional hard assets. Finally, the installation of fibre optic sensing in sewers offers a synergistic opportunity to deploy fibre for broadband and 5G at substantially lower cost and minimal disruption compared to conventional streetworks. This can place sewers at the heart of the smart city.
1. NURON LIMITED, London, United Kingdom
2. University of Sheffield/ Department of Civil and Structural Engineering, Sheffield, United Kingdom
3. University of Sheffield/Department of Mechanical Engineering, Sheffield, United Kingdom