Real-Time Predictive Analytics For Smarter Smart Water Infrastructure
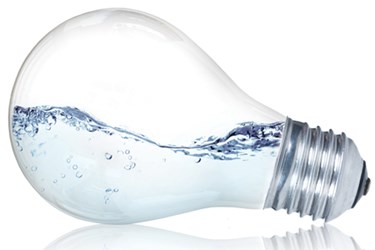
By Sam Hatchett, principal, and Jim Uber, Ph.D., principal, CitiLogics
Are you confused by your smart water system? New tools help utilities make sense of data and finally realize the potential of smart water infrastructure.
Smart water concepts can seem confusing, at least when it comes to our sprawling buried infrastructure systems. Vendors and utilities are both trying to figure out where new technologies will make it easier to solve problems. Right now we’re in the land of Big Data, and it can seem that everybody, from SCADA (supervisory control and data acquisition) suppliers to metering companies, is chanting the same mantra — more data, anywhere you want it, for less money. The assumption seems to be: If the answer is to be found somewhere in the data, then more data should equal better answers.
What’s not to like? Better and cheaper sensors, more-efficient networking — these will be key for most any smart water infrastructure system. But they’re just part of a complete solution for solving real operational and management problems, and, like a highway that’s half finished, you might not see much benefit until the job is done. The Big Data or smart water message can be especially confusing for buried infrastructure systems, with design and operational data often stored in disparate SCADA, CMMS (computerized maintenance management software), customer billing, GIS, and hydraulic modeling databases. If you’re not certain to leverage existing data for specific management and operational goals, then adding more data to the mix can seem hard to swallow.
Data Starvation Or Data Indigestion?
Water utilities, at present, seem more plagued by data indigestion than data starvation. Utilities know they need more data and better data. But it’s a tough sell, until they can process those data efficiently and prove they’re deriving value from investments in expanded or improved instrumentation.
Throughout the industry, it’s common to find that the USB drive, the comma-separated-value (CSV) file, and the spreadsheet have been used as a stand-in for the “data integration platform.” Take, for illustration, a data flow example from the hydraulic modeling arena — one that we know well. Crucial operational data are in the SCADA historian, but always need filtering or other transformations and usually have tag names that are different from their corresponding model identifiers. These data are batch-exported to a CSV file, imported into a spreadsheet, organized and transformed into something that has meaning for the infrastructure — like a water usage pattern — and then imported into modeling software. This process is repeated, as a series of one-off exercises, often by outside consultants. It’s no wonder that hydraulic models are calibrated to data, on average, once every five to seven years.
Utilities can no longer afford such wasteful data management practices. We can do better, and when that happens, it will help clear the path for high-value, smart water infrastructure technologies. Data integration and management tasks need to be simple, intuitive, and meaningful. With that as a starting point, utilities will make efficient use of the substantial data sources they have today and begin to think about tomorrow.
Real-Time Predictive Analytics
“Real-time predictive analytics” can be thought of as distinct from the Big Data techniques borrowed from application areas such as finance, using unspecified methods or purely statistical “black-box” models and indelicately applied to water infrastructure. Instead of a one-size-fits-all approach, real-time predictive analytics fuses operational data streams with information about unique utility infrastructure, creating a fundamentally sound scientific basis for predicting infrastructure performance.
We’re taught in school that the scientific method requires a hypothesis to predict, quantitatively, the results of new observations. Real-time predictive analytics puts this paradigm in motion — predictions of infrastructure behavior produced and updated automatically from real-time operational data. The hypotheses are proven physical laws — conservation of mass and energy — and detailed infrastructure specifications, instead of fragile statistical correlations. Complete predictions of flows, pressures, tank levels, and water quality are produced throughout the infrastructure network, evolving over time so that pump statuses, control valve settings, and water demands reflect actual system operation.
The scientific method also insists that predictions are compared to observations. Real-time predictive analytics are compared continuously to observations in the SCADA record, identifying out-of-bound conditions. The next generation of predictive analytics software will monitor these conditions as sentinels of infrastructure failure and provide the tools to identify small problems before they turn into big ones.
The Future Is Now: Next Generation Software Systems
The industry is moving quickly to address important data integration issues and bring real-time predictive analytics into mainstream utility practice as a foundation for smart water infrastructure. Turnkey configuration processes will eliminate custom software development and lower implementation costs by supporting flexible and robust methods of data access, filtering, and integration with infrastructure elements. Real-time predictive analytics will be generated automatically, using either local or cloud-based data processing, and served to the end user via Web-based, interactive dashboards on desktop, tablet, or mobile platforms. This is happening now with the CitiLogics’ Polaris analytics system, which is a single solution that collates all of these functions and is based on the official USEPA Epanet-RTX real-time analytics engine.
Using such next-generation predictive analytics technologies, utility operators will practice achieving optimization goals for pressure, leakage, energy, and water quality management. Just like a pilot uses a flight simulator, utility engineers will rely on accurate infrastructure models that are continuously updated through a persistent connection to the operational record. Likewise, utility managers will review automated dashboards and periodic reports showing trends in important management goals and integrate those with past and future asset management decisions.
Early adopters of real-time predictive analytics technology are already demanding more than new sensors; they are expecting a flexible data integration platform with a real-time predictive ability that leverages their past investments in data and modeling technologies. Their experiences will benchmark the benefits from a smarter smart water infrastructure based on physics-based, real-time predictive analytics — and pave the way for the broader utility industry.
About The Authors
Sam Hatchett is a principal of CitiLogics and brings research-level engineering expertise and creative mathematical- and physics-based insights into designing models and building tools for large water distribution networks.
Jim Uber, Ph.D., is a principal of CitiLogics, a software technology firm located in the metro Cincinnati area. CitiLogics developed Polaris, a real-time, SaaS predictive analytics suite of tools for the water utility sector.