New-Generation AI Overcomes Barriers To Proactive Leak Detection Adoption
By Emma Quail
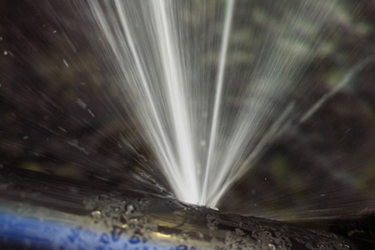
An estimated 90% of leakage never shows above ground,¹ but adoptable technologies are challenging our accepted wisdom on economic levels of leakage (ELL).
Climate change and the prospect of increased regulation are prompting many forward-thinking U.S. utilities to reexamine their attitudes to water leakage.
And it’s difficult to ignore. Water main breaks make daily headlines, due to collateral damages and risks to public health and safety.
Tapping into the world’s last best source of new water is the most obvious solution to water scarcity, and the utilities that grasp the concept first will reap the most benefits both financially and operationally.
In fact, according to researchers in California,2 reducing leaks may already be a cheaper way for most median utilities to save water than even conservation campaigns and rebate programs.
For large utilities, the potential rewards are even greater. Reducing leaks reduces the need for large capital investment into processes such as desalination or water reuse which, ironically, make leakage even more unsustainable. If leaks are barely acceptable when a gallon of water costs under a cent, how will a water utility justify rate increases when it tops $2?
Phone app showing a large leak result and graphs
So why aren’t all water utilities investing in a water loss recovery program when water scarcity and aging infrastructure will not support future demand?
Barriers To Leak Detection
Although benchmarking non-revenue water (NRW) as a percentage is not a reliable performance indicator and doesn’t reflect all the physical losses through leakage, the cited 16% national average of NRW as reported by the U.S. EPA is still alarming. No other industry would find it acceptable to bleed so much retail product and revenue.
A big problem is that many U.S. utilities are challenged internally with a deficit in dedicated leak detection resources and budget restraints.
This is not their fault. The U.S. water sector is facing critical and worsening staff shortages.3 The fear is that this will diminish the knowledge base, and the leak detection discipline will be the loser in the battle to attract and retain new talent.
Budgetary restraints have also been aggravated by a shift in consumption patterns and billed revenues after COVID. While water utilities are getting back on their feet, the imperative is to provide a reliable and safe drinking supply. Leak detection may be perceived as a distraction and an unwelcome expense.
FIDO Bug sensor glowing within a low-pressure plastic network fitting in Bangkok, Thailand
The absence of nationwide water loss control reporting means that, currently, only around half of U.S. states must account for their finished water. A one-size-fits-all approach to enforcement may not deliver the best outcomes for all utilities (California researchers again2), but as a sector we do need a consensus on what should be done. A reactive approach to leak detection, even one with ad hoc acoustic leak detection equipment to address known leaks or an annual system survey, is not sustainable and is labor-intensive. We need to get smarter.
In-hand look at the Bug sensor
Disruptive Technology Offers New Answers
Overcoming these challenges will take more than piecemeal improvement in conventional processes and technologies. Fortunately, a new generation of solutions designed with accurate artificial intelligence (AI) has emerged that minimizes labor requirements, the need for high-skilled employees, and CAPEX restrictions offers revolutionary new levels of accuracy based on real life data.
What makes AI particularly exciting is how it allows utilities with no current proactive or digitized capabilities to set up a complete systemwide, end-to-end smart leak detection program almost overnight. This type of long-term proactive approach will almost guarantee reductions in NRW.
New AI business models based on data as a service (DaaS) now include simple, low-skill, no-calibration, acoustic sensors that also act as accurate in-field correlators and ground microphones to pinpoint leaks, as well as repair validators for evidenced revenue assurance. This upends the need to invest heavily in new equipment before seeing returns.
Continuous Adaptation To Sector Challenges
But the real value of machine learning (ML) technology lies in its ability to constantly learn from actual field data to reduce false positives and provide new kinds of actionable insight.
Leak-sizing4 is a great example of this. Not only has AI delivered accurate leak/no leak results from any source on any material, but it has also been trained to listen for leak size using acoustic files. This previously unavailable insight lets managers prioritize investigations on the leaks that matter — and that’s not always the large ones. As we know, multiple small leaks left undetected can result in pipe failure and be cumulatively more expensive. But ongoing visibility of where leaks are and how they’re changing over time means being able to take informed action when the timing is right and is a smarter allocation of not only money but also limited resources.
Armed with a heatmap of leaks, next-generation acoustic technologies like this are really taking the leak detection industry to another level. Adoptive utilities have halved their overall leak run time just by concentrating on their large leaks. With the imminent addition of remote control and monitoring using the Internet of Things (IoT), managers will remove even more human input from the process, allowing skilled talent to be deployed to where it delivers most value.
References:
- https://www.adb.org/sites/default/files/publication/27473/reducingnonrevenue-water.pdf
- https://cwee.ucdavis.edu/leaks-an-untapped-opportunity-for-water-savings/
- https://www.epa.gov/sites/default/files/2020-11/documents/americas_water_sector_workforce_initative_final.pdf
- https://www.fido.tech/fido-ai/leak-sizing/
About The Author
Emma Quail has more than 20 years’ experience in non-revenue water practices and methodologies in the U.S. and currently is U.S. director of sales at FIDO Tech Ltd., a pioneering software company applying AI in the global water sector.