Machine Learning And The Future Of Water Quality Monitoring
By Andrew Salveson, PE, Kate Newhart, PhD, and Kyle Thompson, PhD, PE
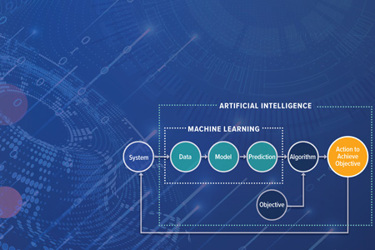
Aided by “soft sensors,” machine learning is revolutionizing monitoring and powering real-time predictions.
Machine learning (ML), a branch of artificial intelligence, is transforming how we monitor and manage water quality. At the forefront of this innovation are soft sensors — not physical devices, but intelligent algorithms that predict slow or expensive-to-measure water quality variables using readily-available data. This breakthrough is reducing monitoring costs and enabling more adaptive treatment processes.
Carollo is pioneering the application of these technologies in water treatment facilities across North America. In this article, we explore two case studies that showcase how ML is reshaping water quality monitoring in potable water reuse systems.
Predicting Total Organic Carbon In Virginia
Imagine being able to predict water quality faster than traditional methods allow. That’s exactly what the Hampton Roads Sanitation District (HRSD) has achieved at the SWIFT Research Center in Virginia.
Total organic carbon (TOC) is a critical parameter for controlling ozone dosing in carbon-based reuse systems. Typically, TOC is measured less frequently than ozone levels, which could lead to less responsive control. Our solution? An ML-powered soft sensor for TOC.
Using three months of historical data from HRSD’s SWIFT Research Center, a 1-MGD carbon-based reuse demonstration facility, Carollo developed a model that predicts TOC levels with remarkable accuracy. A boosted trees (bstTree) model outperformed the last-known value — a linear model with a root mean square error (RMSE) of 0.709 mg/L — by achieving an RMSE of 0.349 mg/L.
The model’s success was based on a comprehensive data set that included measurements at five-minute intervals for 37 water quality and operational variables. Our team extracted 749 TOC measurements and paired them with predictive features such as UV transmittance, pH, and ammonia.
This translates to more precise and responsive ozone dosing, which could lead to significant energy savings and more effective water treatment.
Tackling NDMA In California
Our second case study highlights Las Virgenes Municipal Water District’s (LVMWD’s) Pure Water Demonstration Facility in California, where Carollo faced a different challenge: monitoring N-nitrosodimethylamine (NDMA), a critical disinfection byproduct in potable reuse systems.
NDMA levels can drive UV dosage requirements in advanced oxidation processes downstream of reverse osmosis (RO). Without real-time NDMA sensors, UV doses are typically set conservatively, based on maximum historical concentrations. This leads to unnecessarily high energy use; therefore, Carollo developed an ML-based soft sensor for NDMA.
Using a dataset of 162 NDMA measurements from Orange County Water District’s (OCWD) Groundwater Replenishment System, Dr. Kate Newhart of Oregon State University created a random forest model that predicts NDMA concentrations with an RMSE of 3 nanograms (ng)/L using measurements recorded every three hours over three weeks. Predictive features included ammonia, pH, turbidity, total chlorine, and pressure. As with HRSD, our team developed the ML models using open-source R programming, a powerful tool for statistical computing and data visualization.
Predicted versus measured values of settled TOC for HRSD’s SWIFT Research Center bstTree ML model, showing the accuracy for the (A) training set and (B) testing set.
Implementing UV dosage adjustments based on the predicted NDMA concentrations at OCWD would have resulted in less than 10% energy savings. The average NDMA post-RO was already low compared to the target for groundwater augmentation potable reuse. However, the water district would be held to a lower NDMA target for surface water augmentation potable reuse. Assuming the same model accuracy and starting NDMA concentrations, we estimated the reduced UV energy consumption at LVMWD could be 26%. Incorporating safety factors based on model uncertainty could still achieve 13% energy savings.
Predicted versus measured values of NDMA before UV/AOP at OCWD’s Groundwater Replenishment System.
Therefore, Carollo is transferring this model to LVMWD’s demonstration facility and collecting extensive new data to enhance the model’s efficacy. This includes a comprehensive data collection effort spanning April 2024 to February 2025, and using approximately 200 NDMA samples from RO permeate, with daily sampling at random times to capture daily and seasonal variations. This data set will further refine and validate the NDMA ML model, which could lead to even greater energy savings and treatment efficiency.
A comparison of predicted UV dose requirements for NDMA treatment for the Las Virgenes-Triunfo Pure Water Facility showcases the potential for energy savings with safety factors for the max error observed.
Looking Ahead
These advancements highlight the transformative potential of machine learning and real-time sensor technology in optimizing water treatment processes. By harnessing the power of AI, we’re creating smarter, more efficient, and more sustainable water systems for the communities we serve.
Acknowledgements
The Water Research Foundation funded the TOC soft sensor study as part of Project 5129, “Demonstration of Innovation to Improve Pathogen Removal and/or Monitoring in Carbon-Based Advanced Treatment for Potable Reuse.” The National Alliance for Water Innovation funded the NDMA study under DE FOA 0001905 as part of Project 5.17, “Data-Driven Fault Detection and Process Control for Potable Reuse with Reverse Osmosis.”
About The Authors
Andrew Salveson, PE, is Carollo’s water reuse chief technologist and has received innovation awards from the International Water Association, the California Water Environment Association, and the WateReuse Association, the latter of which pertained to implementation of machine learning for purified recycled water treatment systems.
Dr. Kate Newhart is an assistant professor of Environmental Engineering at Oregon State University. Her research focuses on the development of statistical and machine learning models for fullscale water and wastewater treatment, water reuse, and resource recovery facilities.
Kyle Thompson, PhD, PE, is a senior technologist in Carollo’s water reuse technical practice group and the firm’s national PFAS lead. He has performed numerous research projects, including developing machine learning-based alert systems for drinking water and reuse, and screening chemicals as performance-based indicators or passthrough hazards in reuse.