Johns Hopkins Research Makes Waves With Better Flood Damage Forecasting
By Lisa Ercolano
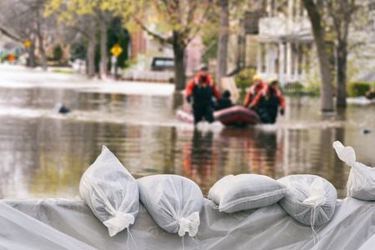
Natural disaster risk modeling provides a reliable and affordable way for governments to estimate expected damage caused by rivers overflowing their banks.
In recent months, rivers overflowing their banks have caused property damage and loss of life in France, Italy, Mexico, and South Africa, underscoring the increasing perils of climate change.
To prepare for such floods, governments deploy mathematical models. However, due to time constraints and lack of data, these models sometimes incorporate "off-the-shelf" damage calculations based on previous unrelated floods. Unfortunately, these predictions are often inaccurate, leading to inadequate interventions and failing to sufficiently protect property and human life.
A Johns Hopkins expert on natural disaster risk modeling has developed a reliable and affordable way for governments to estimate expected damage from riverine floods—those caused by rivers overflowing their banks. This new method not only provides users with step-by-step instructions but also measures and assigns numerical values to the level of uncertainty in individual flood damage forecasts, giving governments a clearer picture of how reliable their predictions are.
"The bottom line is that accurate predictions are crucial to the safety and well-being of people and property. If a government acts based on inaccurate information, its preparation can be off by orders of magnitude, with very serious results," said Gonzalo Pita, an associate research scientist in the Whiting School of Engineering's Department of Civil and Systems Engineering and director of its master's degree program in Systems Engineering, as well as an instructor in the Johns Hopkins Engineering for Professionals' civil engineering program.
The study appears in The International Journal of Disaster Risk Reduction. It builds upon work that previously appeared in The Journal of Hydrology.
In the new study, Pita first investigated the accuracy of using expert opinion alone to estimate and predict flood damage. He surveyed multiple authorities and simulated thousands of expert surveys in numerous combinations to analyze how the composition of the expert team influences prediction accuracy.
"What I found was a variability in accuracy of between 10% and 46% among experts, which is a wide range," Pita said. "I also learned that accuracy was enhanced by adding additional expert voices, rather than simply adding more questions to the survey."
Pita then tackled the issue of "damage functions," a fundamental component of natural disaster risk simulations. A function refers to the mathematical relationship between two variables—in this case, a depth-damage function describes the correlation between the depth of flood waters and the level of damage it causes, as in "1 foot of flood water in a home causes $10,000 in damage." According to Pita, creating these functions accurately is out of the reach of many governments.
"It is a very expensive process in terms of money and time, and countries sometimes may not have the expertise, time, or data to develop them within an acceptable timeframe. So with this method, these functions can be built inexpensively but with a useful level of accuracy that governments can use provisionally until they get better data that will enable them to generate more accurate functions."
The new approach works by helping quantify the uncertainty in experts' predictions by assigning weights to each expert, resulting in a more detailed analysis of the uncertainties involved. The result is a method that Pita expects to be "very useful" for flood modelers and influencing preparedness policy.
"These types of insights could inform policy directly and indirectly, from enabling smarter zoning laws and budgeting for asset maintenance to designing disaster insurance programs. All of this is to say that better flood damage data and predictions have the potential to have far-reaching benefits," he said.
Republished with permission from Johns Hopkins University.