Applying Data-as-a-Service To The Wastewater Sector
By Amir Cahn
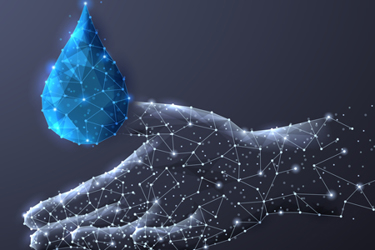
A major concern with real-time monitoring networks is the accuracy and reliability of data. In 2017, the Smart Water Networks Forum (SWAN) surveyed 23 global water utilities about their Big Data management practices as part of a Water Research Foundation (WRF) study, including their barriers to adoption. The results are shown below:
Impediment Factors On Big Data Analysis Adoption Within Utilities
Based on these survey results, “Data quality” and “Lack of talent to create and run Big Data processing and analytics on an ongoing basis” were identified as the greatest impediment factors, followed by “Lack of talent to implement Big Data” and “Data security”. One potential change agent to addressing these pain points is the “Data-as-a-Service” (DaaS) model, an outsourced approach to data collection, delivery, and verification.
What Is DaaS?
DaaS is an innovative business model transforming the way organizations gather, share, and interpret data by accessing data on demand. In his book “Data as a Service: Framework for Providing Re-Usable Enterprise Data Services”, Sarkar defines DaaS as “a framework for designing and developing a set of reusable data services that are designed based on enterprise level standards.” In recent years, DaaS has gained considerable momentum as enterprises across all sectors are moving towards a service-orientated architecture. In essence, DaaS enables users to only pay for the final analytics they wish to receive instead of purchasing and maintaining the equipment themselves. Thus, there are no sunk costs for hardware, data collection, storage, or support with these risks remaining with the data provider. Additionally, DaaS relieves the obstacles involved in training and retaining staff to oversee the operational status of a network. Any type of service involves providing a clear value to customers and facilitating successful outcomes the customer wants to achieve, while managing associated risks. The below 2015 figure from Sarkar displays the key “service” aspects of DaaS:
As described by van Vugt and Jacobsen, there are also notable DaaS challenges, such as concerns over data ownership, data security, and affordability. However, one of the main features of DaaS is that the cost of data collection, cleansing, and analytics is known, making it easier to forecast budget expenses and plan ahead.
Below are a few guiding principles of DaaS, according to Sarkar.
Architecture not technology. DaaS is an architectural framework, beyond a mere technology or application. Its underlying foundation is typically based on the concept of service reuse, enabling users to utilize common, standardized services over the web, the cloud, and related technology for multiple purposes within an organization.
Focus on data quality. For any DaaS service provider, the quality of published data is the primary strategic asset that distinguishes them in the eyes of their service consumers. Therefore, it should be viewed as a key differentiator that must be exploited to drive market share by the data provider. The information fed to DaaS subscribers needs to be consistent, timely, and accurate and meet all the SLA (service-level agreements) specified by business stakeholders with regards to quality and fitness for use.
Data governance challenge. Data governance is often the most challenging aspect of a DaaS program due to the high degree of coordination required to gain consensus among multiple stakeholders on major governance issues. This is impacted by several items including local data laws (e.g., if the data must be encrypted), the support of data quality assurance, security and privacy compliance, data classification, information lifecycle, and auditing features that a DaaS system can support. Anyone considering a DaaS program should be aware that data governance is a critical success factor to the long-term growth and sustainability of data services across the organization.
Within the water sector, DaaS is more commonly applied to wastewater operations with utilities outsourcing the operation and maintenance (O&M) of different services to outside private companies, such as for total wastewater system operations, combined sewage overflow (CSO) monitoring, water quality monitoring, and industrial pollution detection. To provide the required data, the data provider is responsible for acquiring and maintaining the necessary equipment to measure, collect, store, and transmit data to deliver reliable results. In different cases, a utility may choose to purchase the equipment themselves (e.g., flow sensors, level sensors, remote stations), rent the equipment, or only pay for the data they wish to receive. As a relatively new business model for the water sector, there is limited existing information on how DaaS can impact utility operations and improve customer and environmental outcomes.
Next Steps
Despite several successful utility case studies for DaaS within the wastewater sector, there are still several unknowns, such as the questions listed below:
- What are the main utility motivations to implement DaaS? What are the biggest barriers?
- Is DaaS only suitable for utilities that do not have the capacity to install, operate, and maintain their own network?
- How do utility DaaS practices compare across different wastewater applications, as well as across different sectors?
- How does DaaS improve the efficiency of utility O&M?
- Do DaaS utilities have improved regulatory compliance compared to non-DaaS utilities?
- Do DaaS utilities prefer to just acquire data, report summaries, or also predictive insights?
- What makes a utility an appropriate fit for DaaS?
Over the course of the next 6 to 9 months, I will investigate these questions by carrying out (1) a global utility survey and (2) an in-depth study of select utilities using DaaS for different wastewater applications to determine best data management strategies, data quality procedures, contract preferences, regulatory performance, and more. Each of these studies will be available for free to SWAN members.
For more information and to actively participate in this research, please contact Amir Cahn at amirc@swan-forum.com.
Amir Cahn is the executive director of the Smart Water Networks Forum (SWAN), the leading global hub for the smart water and wastewater sectors.