AI For Industrial Process Control (Part 2): Model Predictive Control Deep Dive
By Thouheed Abdul Gaffoor
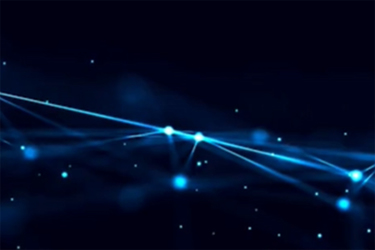
So far, we’ve covered the fundamentals of process control. We reviewed how PID controllers work, discussed their limitations and introduced the concept of predictive control. In this article, we’ll explore how MPC controllers work in more detail.
Model Predictive Control: Recap
Predictive Control operates by performing dynamic, real-time optimization to generate control actions that are adaptive to process disturbances and are compliant with user-specified constraints. Examples of process disturbances include changes in raw material quality, environmental conditions (i.e. precipitation), and upstream operations.
Meanwhile, examples of constraints include regulatory limits or maintenance requirements. MPC allows operators to run their processes more efficiently by operating much closer to constraints than would be possible with conventional reactive controllers in order to save on costs or increase production. This is particularly useful for complex, nonlinear systems such as bioreactors or distillation columns where the impact of tighter controls can yield substantial cost savings.
Get unlimited access to:
Enter your credentials below to log in. Not yet a member of Water Online? Subscribe today.